The KIDMM Community's 'MetaKnowledge Mash-up'
About KIDMM
The British Computer Society [1], which in 2007 celebrates 50 years of existence, has a self-image around engineering, software, and systems design and implementation. However, within the BCS there are over fifty Specialist Groups (SGs); among these, some have a major focus on ‘informatics’, or the content of information systems.
At a BCS SG Assembly in 2005, a workshop discussed shared-interest topics around which SGs could collaborate. Knowledge, information and data management was identified as a candidate. This led to a workshop in March 2006 [2], from which emerged KIDMM (Knowledge, Information, Data and Metadata Management. About a third of subscribers are from communities outside the BCS [3]. The MetaKnowledge Mash-up conference was billed as a ‘sharing and thinking day’, the name reflecting KIDMM’s aspiration to put together, in new ways, knowledge that different communities have about managing knowledge, data and information.
‘Handles and Labels’
The day was chaired and introduced by Conrad Taylor, who acts as co-ordinator for KIDMM. Conrad identified three ways in which we add ‘handles and labels’ to information within computer systems, to make it easier to manage. In a database, data obtains meaning from its container: a number is made meaningful by being placed in a ‘date of birth’ field, or a ‘unique customer ID’ field, etc. Explicitly documenting the meaning of fields and their inter-relationships becomes important when databases need to be merged or queried.
Since the 1970s the term metadata has been used within the data management community to refer to information that describes the allowed content types, purposes and so on of database fields and look-up relationships. This meaning may surprise librarians and information scientists, who started using ‘metadata’ to mean something different in the early nineties (Lorcan Dempsey of UKOLN being perhaps to blame). As a result, any conversation about metadata within the KIDMM community, which bridges management of textual information and data, has to include disambiguation about which of these meanings is intended [4].
Information in text form tends to lack ‘handles’. There are exceptions: mark-up languages such as SGML and XML allow entities within text to be given machine-readable semantic contexts. Military systems documentation has used SGML/XML structure in support of information retrieval for a couple of decades. Failing that, we rely on free text search, with all its frustrations.
The third ‘handle-adding’ approach which Conrad described is the attaching of cataloguing data labels, or ‘metadata’ in the librarians’ sense of the word. This may live outside the information resource, as in a library catalogue, or be embedded in the resource.
Dublin Core is a well-known starter-kit of metadata fields. One troublesome Dublin Core field is the Subject field: here we bump into problems of classification. Conrad called on Leonard Will to give the conference a briefing on the issues later in the day.
Information Retrieval Today: An Overview of Issues and Methods
Tony Rose, of the BCS Information Retrieval SG, offered us Wikipedia’s definition of information retrieval: search for information in documents, or the documents themselves, or metadata which describes documents.
The ‘naive science’ view of how search engines like Google function is that they understand what users want to find. However, all search engines do is count words and apply simple equations; they measure ‘conceptual distance’ between a user’s query and each document in their database. Authors of documents express concepts in words as do searchers, in the search terms they choose. The central problem in IR is whether a good conceptual match can be found between the searcher’s terms and the author’s.
To process searches, a search engine must represent the search terms and author’s terms internally. The usual ‘bag of words’ approach treats every document as a collection of disconnected words. The similarity of the contents of the ‘bag’ to the search terms is calculated by set theory, algebraic or probabilistic methods, the algebraic approach being the most common.
In evaluating the effectiveness of information retrieval systems, two key terms are ‘precision’ and ‘recall’. A search is insufficiently precise if it brings back lots of irrelevant documents, and scores poorly on recall if many documents relevant to the searcher’s query are not retrieved. Unfortunately improvements in precision are usually to the detriment of recall, and vice-versa.
What makes search hard? The bag-of-words model has the weakness of treating ‘venetian blind’ and ‘blind Venetian’ as equivalents. Words shouldn’t be treated so independently in documents; word order is important; and relevance is affected by many structural and discourse dependencies and other linguistic phenomena. In many search engines, the database is reduced in size by excluding words such as the, and or of (the ‘stop words’ approach). The trouble is, these are useful function words: by excluding them, much linguistic structure is thrown away.
Then there is the problem of named entity recognition. ‘New York’ as an entity is destroyed by the bag-of-words approach. One solution is to add a gazetteer of geographic entities. One could do the same for people’s or organisations’ names.
Moving from syntax to semantics, matching concepts presents greater challenges. It would be nice if a system would recognise car and automobile as synonymous. Some systems use a thesaurus, but it can be problematic to decide at what level of abstraction to set equivalence (in some use-cases car and bus might be equivalent, in other cases not), and building thesauri always involves a huge amount of human editorial input.
The flip side of concept matching is the problem of disambiguation: there are at least three meanings for the word ‘bank’. One should have the riverside distinguished from the financial institution and the aerobatic manoevre.
If search is so hard, how come Google gets it right so often? Google’s originators had the insight that on the Web there is another index of relevance: the links to document being indexed. The more links point to a page, the more highly it is rated by users.
Data Mining, Text Mining and the Predictive Enterprise
Tom Khazaba of SPSS focused on data mining issues in commercial and government contexts. Most large organisations may derive insights of practical use by applying an analytical process to the data they keep. ‘Data mining’ refers to the core of that analytical process. ‘Predictive analytics’ is a term encompassing the broader context of use: it ‘helps connect data to effective action by drawing reliable conclusions about current conditions and future events’.
In business, a common goal of predictive analytics is to get customers more efficiently and cheaply; identify services to sell to existing customers, and avoid trying to sell them things they don’t want. By analysing why customers close accounts, banks could learn how to retain customers. The second largest group of business applications of predictive analytics is in risk detection and analysis, e.g. detecting fraud and suspicious behaviour. Predictive analysis has many uses right across government.
Describing the technology, Tom introduced a commonly used algorithm called a ‘decision tree’. In data mining, a decision tree breaks a population into subsets with particular properties, and can do so repeatedly, sifting data to map from known observations about items, to target categorisations with predictive use. Clustering algorithms, which find natural groups within data, are often used in statistical analysis; in data mining they are commonly used to find anomalies.
In summary, data mining algorithms discover patterns and relationships in the data, and from this they generate insight into things one had not noticed before, which can lead to productive change. They also have predictive capability.
SNOMED Clinical Terms: The Language for Healthcare
Ian Herbert Vice Chair, BCS Health Informatics Forum
SNOMED-CT stands for ‘Systematized Nomenclature for Medicine Clinical Terms’, and is the result of the 2002 merger of the American SNOMED Reference Terminology (RT) with the UK National Health Service Clinical Terms, Version 3. The latter was developed from the ‘Read Codes’ made commercially available by UK physician Dr. James Read, and purchased by the NHS in 1998.
Ian introduced SNOMED-CT as ‘a terminological resource that can be implemented in software applications to represent clinically relevant information reliably and reproducibly.’ It has been adopted by the NHS Care Records Service.
The purpose of SNOMED-CT is to enable consistent representation of clinical information, leading to consistent retrieval. In direct patient care, clinical information documents people’s health state and treatment, through entries in electronic patient records. Decision support information assists health professionals in deciding what action is appropriate.
Because people move between different health providers (GP, hospital, specialist clinic), there is a need for semantic interoperability between the information systems of all providers: meaning must transfer accurately from system to system. A controlled terminology will help deliver this.
Nobody believes that all clinical information can be structured and coded; there will always be a role for diagrams, drawings, speech recordings, movie clips and text. However, SNOMED enables consistent ‘tagging’ of information about individual patients, plus knowledge sources such as drug formularies.
How will we know when SNOMED-CT is successful? Ian closed with a quotation from Alan Rector of the Medical Informatics Group at the University of Manchester:
‘We will know we have succeeded when clinical terminologies in software are used and re-used, and when multiple independently developed medical records, decision support and clinical information retrieval systems sharing the same information using the same terminology are in routine use’ [8]
Geospatial Information and Its Applications
Dan Rickman, Geospatial Specialist Group
Geospatial information systems (GIS) ‘[allow] the capture, modelling, storage and retrieval, sharing, manipulation and analysis of geographically referenced data.’ At the heart of any GIS is a database, containing objects with attribute data. In the past, GIS has been ‘sold’ with a more pictorial view, with the map at its heart. The more recent trend is to emphasise the data, and say that any map is a spatial representation of what is in the database. This is a paradigm shift in thinking about GIS, which its practitioners are encouraging. Presentation of geospatial information doesn’t even have to be map-based. If you want to know which stores are nearest, search may be spatial, but delivery could be a list of addresses.
Geospatial data used to be a simple join-the-dots model. These days we have concepts of topology, structure, objects etc. GIS requires metadata: at its simplest, a co-ordinate system, without which the co-ordinates would be as useless as a financial database that did not define its currency.
Who uses geospatial data? Examples include central and national government agencies, public utilities, insurance companies (consider flooding!) and health (especially epidemiology). Travel is a large field of application: SatNav of course, multimodal route planning and optimisation of delivery routes.
Historically, much geospatial data has been sidelined in proprietary formats, sitting outside the database, not readily accessible by other systems. There is now a shift towards moving spatial data into mainstream database technology, so spatial data is modelled as a complex data type inside databases.
Dan showed three kinds of GIS representation applied to an urban area: old-style Ordnance Survey Landline ‘join the dots’ mapping; the object-oriented approach, in which each building is a database object with a ‘TOID’ (Topological Identifier); and aerial photography, which is rich in features but hard to interpret.
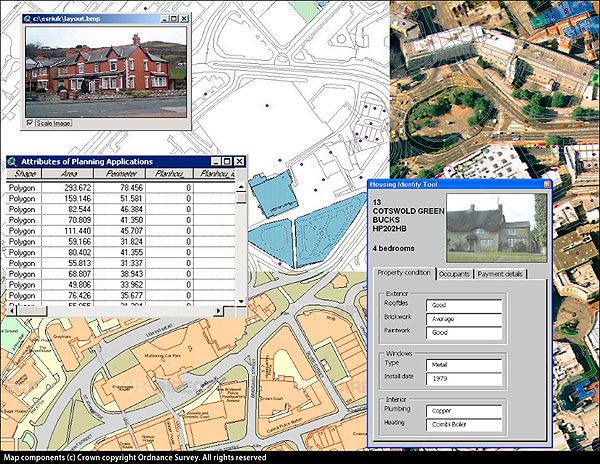
Figure 1: A montage of various types of geospatial data and imagery
GIS databases now support more structure than in the past. An initiative called the Digital National Framework encourages organisations to structure their data with reference to objects, rather than to recapture and duplicate it. The principles of the DNF are:
- Capture information once, use it many times.
- Capture the highest resolution possible, avoiding the need for later re-capture, and improving the potential for data interoperability.
- Where possible, use existing proven standards.
Recently there has been a ‘quest’ to define the BLPU (Basic Land and Property Unit). However, entities one wants to keep track of can be complex. On the Ordnance Survey Master Map, ‘St Mary’s football stadium, Southampton’ is a single unit. The nearby railway station is recorded as multiple objects - main building, several platforms, pedestrian bridge. As for hospitals, there are a number of buildings, and ownership may be complex. Clinics off-site are organisationally part of the hospital but not contiguous with it. How these relationships are described can also change according to requirements for data use.
To make sense of scanned images of maps in GIS, metadata such as the co-ordinate system and projection system used is needed. There is a trend to placing scans in databases as BLOBs (Binary Large Objects). Raster map data is problematic because it requires human interpretation; progress in map-based pattern recognition systems is slow.
The Geospatial SG believes that geospatiality is a field whose time has come. There is greatly increased public awareness, thanks to Google Maps and SatNav. This can only increase in the future as more phones will incorporate GPS, driving a host of location-based services.
Integrating Museum Systems: Accessing Collections Information at the Victoria and Albert Museum
Christopher Marsden
A challenge often faced in data management is when databases, independently developed, have to be integrated together. The problems are not purely technical; the component databases reflect a particular way of looking at their subjects, and there may be organisational politics involved. The Core Systems Integration Project (CSIP) at the V&A is a case in point.
The Victoria and Albert Museum contains about 1.5 million objects. These are inventoried and in some cases catalogued electronically in the V&A’s Collections Information System, based on SSL’s MUSIMS software [10]; this conforms to the MDA’s SPECTRUM standard. In addition, there is a large amount of paper-based documentation about objects in the collections.
There are about 1.5 million items in the National Art Library, plus vast archives. The library database is a standard system supplied by Horizon. There is no database for archives; these are stored as Encoded Archival Description (EAD) XML files [11].
The Museum also holds about 160,000 images of objects in the collections. These are documented in the Digital Asset Management database, and images are available for purchase online.
The information systems for the library, archive and collections are all large, well established, and based on different recognised international standards. The separate standards are of long standing, have been carefully developed, and there are justifiable reasons for the differences. But it does create problems in getting such different systems to work together.
The Museum staff have begun to have larger aspirations about ways in which data owned by the V&A could be used; and it may be regarded almost as a scandal that despite the huge amounts of data the Museum has, the public is unable to access quite a lot of it.
From this starting point, the aims and objectives of the Core Systems Integration Project were:
- to develop a system architecture whereby various applications could access information about objects in the collection through a Virtual Repository, rather than mastering object data locally; and
- to integrate the Museum’s core systems, and remove the dependencies on manual data manipulation inherent in existing practice, thus improving efficiency and accuracy of data delivery.
The Virtual Repository is now in place - and it works. A prototype Gallery Services application exists, serving information and pictures in a way that Gallery staff and the public can use, but it isn’t installed yet. Progress has slowed recently, but Christopher is optimistic that they will be able to push ahead and deliver.
Further information on the development of CSIP
Preservation of Datasets
Terence Freedman, NDAD, The National Archive
The National Archives (TNA) was created in 2003 as a merger of the Public Record Office, the Historic Manuscripts Commission and the Family Records Centre; the Office of Public Sector Information merged with TNA in 2006. The information they look after goes way back - to the Domesday Book!
The Public Records Act 1958 stipulated that government data should be released after 30 years (the ‘30 year rule’). Later the threshold dropped to seven years; thanks to the Freedom of Information Act, much government data can now be accessed immediately. In theory. But in practice, some information is ‘redacted’ - edited out of the record, through a decision of the Lord Chancellor’s Advisory Committee. Thus there are blanks in some released documents.
The National Archives has responsibility for documents, records and datasets. Documents come from the Electronic Document and Records Management Systems of government departments; they have provenance, and various sorts of metadata attached; they certainly have sufficient attached data to make cataloguing them fairly straightforward. But the National Digital Archive of Datasets (NDAD) mostly deals with tabular data - much more problematic.
NDAD acquires ‘born digital’ data, which can be up to 30 years old. That might mean punched cards, paper or magnetic tape. The threat of loss of data through decay is quite pressing in the case of magnetic records. Surrounding information is often missing - what methodologies were used for collecting that data; why it was collected; who collected it; what format it was contained in and how it was encoded.
The aim is to improve access to this data by indexing it. This does work! Type ‘NDAD’ into Google and you will be into TNA’s data in no time at all, promised Terry: type in ‘NDAD badgers’ or ‘NDAD trees’ and you will be into that particular selection of datasets. (Though Richard Millwood later noted that when he tried the latter, Google asked if he had meant ‘dead trees’ instead!)
To make access possible, it is vital to move rescued data in its typical chaotic state through common processes, resulting in a standard encoding; for example, tabular data is converted to CSV files (comma-separated values), and relationships are created between tables, such that they are maintainable from that point on. That done, the original software can be forgotten; it is no longer relevant once data has been freed from those dependencies.
Nothing in the record must change - it is inviolable, even if the original data was wrong. Other agencies, such as the Department for Work and Pensions, or the Office for National Statistics, do correct this data, resulting in highly usable results. But NDAD’s role is strictly preservation.
Recovering information from government departments can be difficult, involving liaison with Digital Records Officers (DROs). NDAD staff acquire the datasets, check them for completeness and consistency, and transport them to the University of London Computer Centre (the contractors) for processing.
Early in the process, materials are copied literally bit-for-bit from the original media onto current media; then the original data carrier is disposed of securely. A further bit-copy is made, and conversion to current encodings and standard formats proceeds from there. ‘Fixity checking’ is performed at frequent intervals to ensure that data has been copied faithfully, and multiple redundant back-ups are maintained via remote storage.
When NDAD acquires digital data created with obsolete software, certain assumptions are made at the start, such as ASCII encoding. Then there is a process of testing against the bit-copy until the record is ‘cracked’ and becomes legible. The target is to shift data into a canonical representation that frees it from dependency on any software.
Access to NDAD data is free. On average there are 55,000 Web page accesses each month; there are 116 million records currently, spread across 400 datasets. Access to data has remained excellent, with only a few hours outage in a year.
Classification
Leonard Will (Willpower Information) is a consultant in information management, with an interest in libraries, archives and museums; also a member of a BSI working party on structured vocabularies for information retrieval (BS 8723).
He told us we must distinguish between making descriptions of documents and providing access points to them. When you retrieve a list of documents, you should be presented with information allowing you to assess which documents are relevant. We should also distinguish between (a) the structured form of data that you need for consistent indexing and (b) information presented about the document once you have found it, which can be free text. Controlled vocabularies are used to bring consistency in indexing, so there is a better chance of matching terms used by a searcher with terms applied by an indexer.
In human (intellectual) indexing, the first step is a subject analysis to decide what the document is about. Having identified the concepts represented in documents, you translate them into the controlled vocabulary for labelling those documents.
In a controlled vocabulary, we define concepts, and give them a preferred term. We may also give them other, non-preferred terms. The preferred term is used as an indexing label, and is to some extent arbitrary. It should be equally possible to achieve access using any non-preferred term. The definition of the scope of a concept - what it means - is best expressed in a scope note. This should say what’s included in the concept, what’s excluded, and what related concepts should be looked for under another label.
Concepts can be grouped in various ways within a controlled vocabulary. In a thesaurus, they can be labelled separately, with relations between them specified. The relations in a thesaurus are paradigmatic - true in any context. For example, the term ‘computers’ may be related to ‘magnetic tapes’. Broader and narrower terms are common forms of relation, e.g. ‘computers’ and ‘minicomputers’.
However, it is not the job of a thesaurus to link terms like ‘computers’ and ‘banking’. Such a relationship is not inherent in the concepts themselves. In dealing with documents about computers and banking, the relationship between the concepts is said to be syntagmatic - they come together in syntax to express the compound concept: ‘computer applications in banking’.
‘In a classification scheme, we build things using concepts which come from different parts of the thesaurus - from different facets, if you like,’ said Leonard. The term ‘facet’ is fashionable, and often mis-used. A ‘fundamental facet’ is a particular category such as objects, organisms, materials, actions, places, times. These categories are mutually exclusive: something cannot be an action and an object at the same time. Facets can be expressed in a thesaurus, and you can only have broader-narrower, or ‘is-a’ relationships between terms within the same facet. But in a classification, we can combine facets together: e.g. banking as an activity and computers as objects, combining to express a compound concept.
When combining facets to create compounds in a classification, you have to do it in a consistent way. Therefore we establish a facet citation order. The Classification Research Group citation order for facets starts with things, kinds of things, actions, agents, patients (things that are operated on) and so on, ending up with place and time. This is an elaboration of the facet scheme proposed by Ranganathan, the ‘father’ of faceted classification; his formula for the citation order was PMEST, for Personality, Matter, Energy, Space and Time.
A classification scheme doesn’t bring things together alphabetically, so it is often necessary to have a notation, such as a numbering scheme, which allows you to sort and maintain things systematically. Otherwise you’d get Animals at the beginning and Zoos at the end; yet because they are related, we expect to find them close together.
This brought Leonard to the difference between the thesaurus approach and classification approach. A thesaurus approach lets you do post-coordinate searching, and find things by particular terms. In post-coordinate searching, you combine concepts after indexing, i.e. at search time. Pre-coordination means that terms are combined at the point of indexing into a compound term.
The value of a classification is that you can browse it usefully. Leonard thought it a pity that in modern online library catalogue systems, we can no longer browse as one used to be able to do in a card catalogue, finding related concepts to either side. Whereas a classification scheme should allow this kind of browsing, a thesaurus is useful for searching. The two are complementary: it is best if you can have both.
All too often we see a user interface with a tiny box, into which we are asked to type search terms, without any guidance about what we can do. Even our advanced interfaces often give insufficient guidance about how to compile a sensible search statement. Whereas Google often says ‘Did you mean X?’, a thesaurus could do much more. It could say, ‘I’ve got this, but I index it under this term; I’ll do the search on that instead,’ or, ‘You’ve searched for this, would you also be interested in searching for the following related terms?’
This kind of interaction is what librarians call the reference interview: for example when somebody asks ‘Where are the chemistry books?’ when what they really want is to find out if the weedkiller they put down their drain is dangerous. People are generally thought to ask for topics broader than they really want, because they are frightened to be too specific. Ideally a search interface should be able to lead the enquirer through that kind of reference interview. A computer can do it in principle; but the systems we have at present do not.
Discussion: Taxonomies and Tagging
Enabling Knowledge Communities
Richard Millwood is the Director of Core Education UK, and a Reader at the Institute for Educational Cybernetics at the University of Bolton. He was formerly the Director of Ultralab at Anglia Ruskin University, where he worked on the Ultraversity project.
Richard is also a consultant to the Improvement and Development Agency (I&DeA). Introducing him, Conrad referred how impressed he and Genevieve Hibbs had been with I&DeA’s knowledge community support systems. They had recommended Richard to lead discussion of how we can build and foster such communities online.
Ultraversity
The Ultraversity degree is a BA (Hons) in Learning Technology Research (LTR). It’s a ‘learn while you earn’ course, with a full-time credit rating, and combines a number of innovations.
LTR is inquiry-based learning, with an action-research methodology. Students focus on improving the work they already do, based in their own work environment. The course is personalised: each student negotiates a study focus with the university to create an individual learning plan. Assessment is by dissertation and presentation of an e-portfolio, assisted by a peer-review process. Most relevant from KIDMM’s point of view is that the Ultraversity system contextualises learning within an online community which offers support and critical feedback, with input from experts and access to an online library.
The LTR course was shaped additionally to give participants skills, knowledge and confidence in the use of online services, equipping them for lifelong learning as online practitioners beyond the point of qualification. Ultraversity provides its students with a safe space, a ‘launch pad’ for public identity online.
Before constructing the Ultraversity scheme, Richard and his Ultralab colleagues had worked on prior projects with teachers such as Talking Heads, the purpose of which was to ensure that head teachers keep up with each others’ learning and skills. The Ultralab team discovered that as these online communities developed in strength -creating spaces in which participants could trust each other - it raised the quality of debate and led to deeper learning [17].
Learning in Ultraversity arises through students expressing ideas, then evaluating these expressions. For this to happen, people must feel safe from ridicule. Students’ prior experience of schooling meant that they did not trust the concept of learning from other ‘ordinary’ people; one student wanted all information volunteered online to be validated by her Learning Facilitator. Over time, she realised her fellow students ‘were extraordinary people, with a wealth of knowledge and especially experience among them.’
I&DeA Knowledge
In January 2007, Richard went to work with Marilyn Leask’s team at the Improvement and Development Agency. I&DeA Knowledge is a ‘one-stop shop’ Web site giving rapid access to background knowledge required to do the job of local government effectively. It pools national, regional and local perspectives; provides a means of communicating with experts in other local authorities; and is a source of authoritative and reliable information. This last point is of interest in relation to the issue of authority: if you work in a local authority, whom would you trust for knowledge about tackling climate change at local level: central government? or the local authority that’s been winning prizes for its climate change policy?
The I&DeA team set about trying to explain to local authorities how the agency can help them learn from best practice. For example: on the I&DeA Knowledge Web site, they pulled together knowledge about climate change from local authorities which are particularly forward-looking about the issue. Richard displayed a page with links to relevant discussions on the site: encouraging sustainable travel behaviour, calculating CO2 emissions, carbon and ecological footprinting for local authorities, and sustainability training materials.
Such discussions are not, strictly speaking, online communities - they are bulletin boards hosted on the system, where people make contributions. These are not contexts in which people form relationships, by and large. Richard repeated the point to hammer it home: Online Community does not equal discussion forums - they are collections of people, not a software facility.
Some resources are centrally produced: toolkits to support a range of actions, and documents to support learning and staff development. But for KIDMM, the I&DeA Communities of Practice are of greater interest. There are about fifty of these. Joining a CoP helps people quickly obtain practical solutions to issues; access cutting-edge practices and thinking; and network to share knowledge and experience. In a CoP, you can share bookmarks, post slides or documents, and otherwise communicate with colleagues in a form that stays ‘on the record’. The I&DeA system also has a wiki functionality, used for developing shared, collaboratively-authored documents.
Richard showed us an event announcement which he had tagged it with keywords. ‘Taggers’ choose their own keywords; but the system displays a list of the most commonly used, so the tagging terms with which people feel most comfortable are rising to the top of the heap.
Conclusions and Aftermath
Outputs from the Mash-up are being made available on the KIDMM Web site, including slides, some audio from talks, and a more comprehensive version of this Report [18]. Several more people have joined the discussion e-list [3], and an e-newsletter service has been set up so that people who want to keep an eye on KIDMM’s activities can be informed on a fortnightly basis [19].
Inspired by Richard Millwood’s advocacy of online community, the new domain www.kidmm.org has been registered, and the aim is to develop there an electronic discussion space eventually open to wider participation. The other major current KIDMM project is the construction of a portable consciousness-raising exhibition, Issues in Informatics, two prototype panels of which were on display at the event.
References
- BCS Web site http://www.bcs.org
- 6 March KIDMM workshop report http://www.epsg.org.uk/KIDMM/workshop.html
- BCS-KIDMM@JISCmail.ac.uk - membership list with affiliations is at http://www.epsg.org.uk/KIDMM/email-list.html
- Conrad Taylor, 2007, Metadata’s many meanings and uses - briefing paper http://www.ideography.co.uk/briefings
- Danny Budzak, Metadata, e-government and the language of democracy. http://www.epsg.org.uk/dcsg/docs/Metademocracy.pdf
- Workshop report Information Literacy, the Information Society and international development http://www.epsg.org.uk/wsis-focus/meeting/21jan2003report.html
- CRISP-DM http://www.crisp-dm.org/
- Alan Rector. 2000. ‘Clinical Terminology: Why is it so hard?’ Methods of Information in Medicine 38(4):239-252; also available as PDF from http://www.cs.man.ac.uk/~rector/papers/Why-is-terminology-hard-single-r2.pdf
- See http://www.openehr.org/
- See comprehensive description of MUSIMS at the MDA Software Survey: http://www.mda.org.uk/musims.htm
- Official EAD site at the Library of Congress: http://www.loc.gov/ead/ See also Wikipedia description at http://en.wikipedia.org/wiki/Encoded_Archival_Description Accessed 25 October 2007.
- See CIDOC Web site: http://cidoc.mediahost.org/standard_crm(en)(E1).xml
- SCULPTEUR - http://www.sculpteurweb.org/ Includes ‘a web-based demonstrator for navigating, searching and retrieving 2D images, 3D models and textual metadata from the Victoria and Albert Museum. The demonstrator combines traditional metadata based searching with 2D and 3D content based searching, and also includes a graphical ontology browser so that users unfamiliar to the museum collection can visualise, understand and explore this rich cultural heritage information space.’
- Seamless Flow Programme http://nationalarchives.gov.uk/electronicrecords/seamless_flow/programme.htm
- See results table for the 2 September 2007 ballot at http://en.wikipedia.org/wiki/Office_Open_XML_Ballot_Results Accessed 25 October 2007.
- See Adobe Labs Web site http://labs.adobe.com/technologies/mars/
- Carole Chapman, Leonie Ramondt, Glenn Smiley, ‘Strong community, deep learning: exploring the link’. In Innovations in Education & Teaching International, Vol 42, No. 3, August 2005.
- Outputs are being posted at http://www.epsg.org.uk/KIDMM/mashup2007/outputs.html
- The KIDMM News e-newsletter can be subscribed to at http://lists.topica.com/lists/KIDMM_news